Edge computing and Machine-to-Machine (M2M) platforms are rapidly converging to revolutionize various industries by enhancing performance, reducing latency, and enabling more efficient data processing. This synergy is vital in modern IoT ecosystems where the demand for real-time data processing and actionable insights is higher than ever.
What is Edge Computing?
Edge computing involves processing data closer to the source of data generation rather than relying on centralized cloud servers. By decentralizing data processing, edge computing significantly reduces the time it takes for data to travel, thus minimizing latency and improving the efficiency of data handling.
M2M platforms facilitate communication between devices without human intervention. By integrating edge computing, M2M platforms can enhance their capabilities, offering quicker response times and more robust data processing.
Enhancing Performance through Edge Processing
With edge computing, data generated by devices is processed locally, allowing for immediate analysis and response. For instance, in a manufacturing setting, edge-enabled M2M platforms can instantly detect and address equipment malfunctions, reducing downtime and improving operational efficiency.
Latency is a significant concern in applications requiring instantaneous feedback. By processing data at the edge, M2M platforms can minimize the time it takes for data to be transmitted to and from a centralized server. This reduction in latency is important for applications like smart grids, where rapid decision-making is necessary to maintain stability and efficiency in energy distribution.
Edge computing helps optimize bandwidth usage by filtering and processing data locally, transmitting only relevant information to central servers. This approach reduces the amount of data sent over networks, minimizing congestion and lowering transmission costs. In remote monitoring applications, for example, only critical alerts or summarized data are sent to the cloud, reducing the overall data traffic.
Improving Reliability and Resilience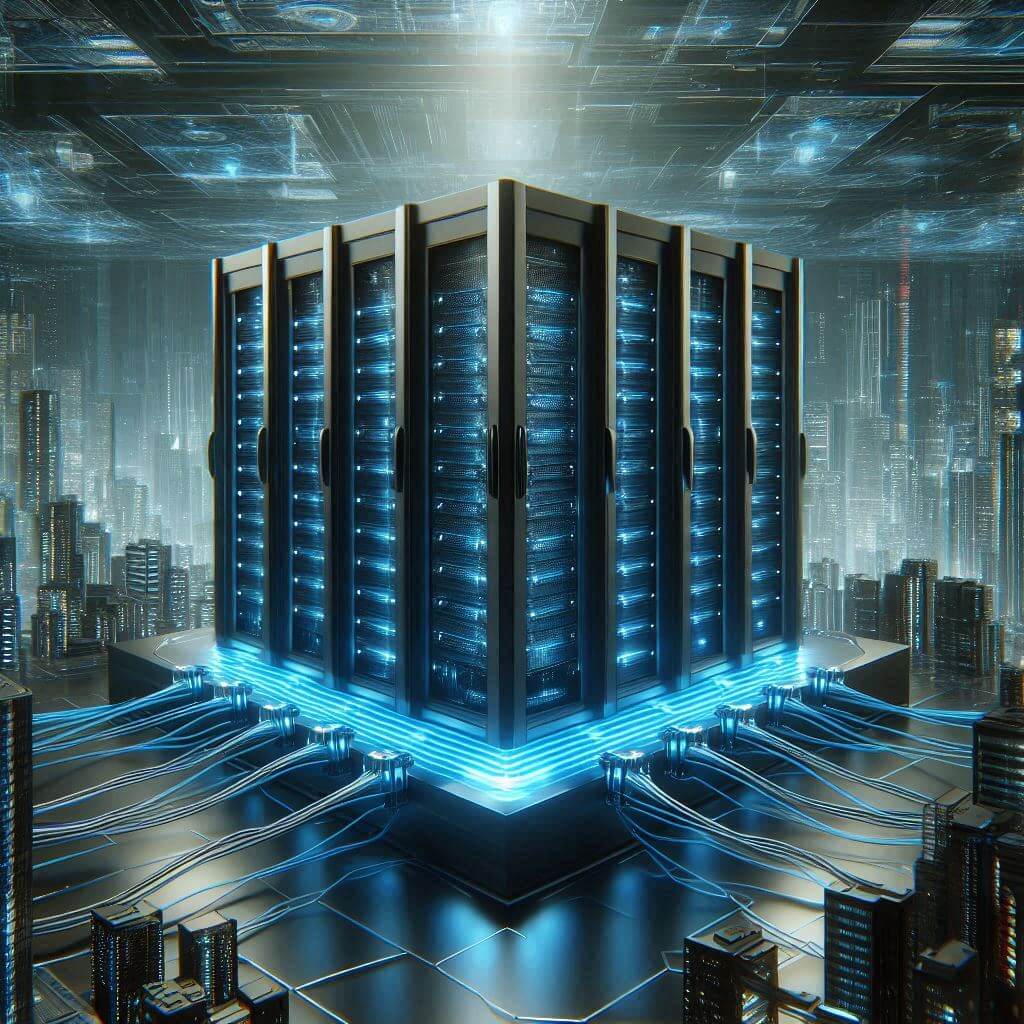
In edge-enabled M2M systems, devices can make decisions based on local data without relying on cloud connectivity. This localized decision-making is vital in scenarios where network connectivity is unreliable or intermittent. For example, in remote oil and gas operations, edge computing allows for continuous monitoring and control, even in areas with poor connectivity.
By processing data locally, edge computing reduces the need to transmit sensitive information over potentially insecure networks. This localized processing enhances security and privacy, as data remains closer to its source. In healthcare, for instance, patient data can be processed at the edge, ensuring compliance with privacy regulations and reducing the risk of data breaches.
Edge computing enables M2M platforms to scale more efficiently. As the number of connected devices grows, processing data at the edge reduces the burden on central servers, allowing the system to handle increased data loads without significant performance degradation. This scalability is main for IoT deployments in smart cities, where thousands of devices generate vast amounts of data.
Practical Applications of Edge-Enhanced M2M Platforms
In industrial settings, edge computing allows for real-time monitoring and control of machinery and processes. M2M platforms equipped with edge processing capabilities can analyze data from sensors and equipment, detect anomalies, and trigger maintenance actions promptly. This proactive maintenance reduces downtime, enhances productivity, and extends the lifespan of equipment.
Wearable devices and medical sensors can process data locally, providing instant feedback to healthcare providers. This immediate response is necessary for managing chronic conditions, detecting health issues early, and delivering personalized care.
In transportation, edge-enabled M2M platforms facilitate real-time vehicle tracking, traffic management, and predictive maintenance. By processing data at the edge, these systems can make immediate decisions, such as rerouting vehicles to avoid traffic congestion or identifying potential vehicle failures before they occur. This real-time decision-making improves efficiency and safety in transportation networks.
Overcoming Challenges in Edge-Enabled M2M Deployments
Ensuring seamless communication and compatibility between diverse devices and systems is crucial. Standardizing protocols and interfaces can help achieve interoperability, allowing different components to work together efficiently.
While edge computing enhances security by keeping data local, it also introduces new security challenges. Protecting edge devices from cyber threats and ensuring secure data transmission are critical. Implementing robust security measures, such as encryption and secure boot processes, is necessary to safeguard the system.
Deploying and managing edge infrastructure can be complex, especially in large-scale IoT deployments. Effective management tools and strategies are necessary to monitor, update, and maintain edge devices. Automated management solutions can help streamline these processes, ensuring optimal performance and reliability.
Future Prospects of Edge-Enhanced M2M Platforms
Combining edge computing with artificial intelligence (AI) can enhance the capabilities of M2M platforms. Edge AI enables devices to perform complex data analysis and decision-making locally, reducing reliance on cloud-based AI. This advancement opens up new possibilities for applications such as autonomous drones, smart agriculture, and real-time video analytics.
The rollout of 5G networks will significantly boost the performance of edge-enabled M2M platforms. With its high-speed, low-latency connectivity, 5G will enhance real-time data processing and communication between devices. This advancement will drive innovation in areas like smart cities, autonomous vehicles, and industrial automation.
As edge computing and M2M platforms continue to evolve, the focus will shift towards advanced data analytics. Leveraging edge analytics, businesses can gain deeper insights from their data, enabling more informed decision-making and optimizing operations further. Enhanced analytics will drive improvements in predictive maintenance, demand forecasting, and customer behavior analysis.
While edge computing reduces the need for constant cloud connectivity, integrating edge and cloud systems will remain important. Edge-to-cloud integration allows for seamless data transfer and analysis, ensuring businesses can leverage the strengths of both edge and cloud computing. This hybrid approach will enable more comprehensive data insights and better resource utilization.